From AI to Quantum: The Evolution of Cutting-Edge Tech
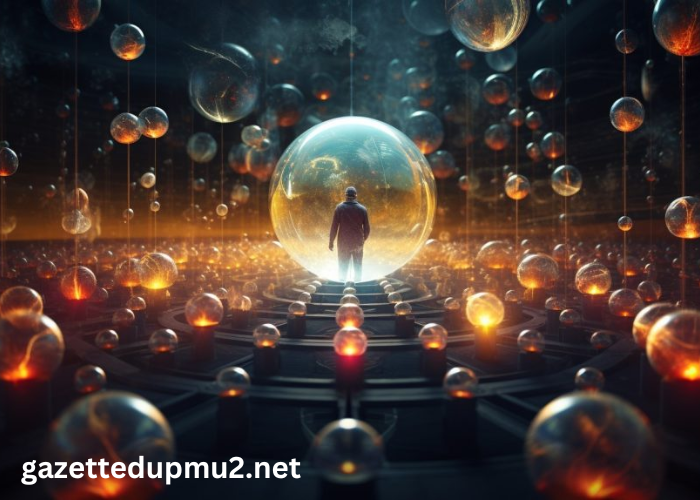
In the ever-accelerating world of technology, where innovation is a relentless force, two fields stand out as pioneers: Artificial Intelligence (AI) and Quantum Computing. These are not just trends; they are revolutions, reshaping our understanding of computation, problem-solving, and the very fabric of reality itself. As we delve into the evolution of these cutting-edge technologies, we witness a journey from the abstract realms of mathematics and physics to the tangible, transformative applications that promise to redefine industries and change our lives.
The Rise of Artificial Intelligence
Artificial Intelligence, once relegated to the realm of science fiction, has come to permeate our daily lives in ways we may not even notice. It’s in our smartphones, our social media feeds, and the voice-activated devices that control our homes. But the journey from Alan Turing’s theoretical Universal Machine to today’s sophisticated AI systems has been long and complex.
The Birth of AI: Turing and Beyond
The roots of AI can be traced back to the mid-20th century when British mathematician and logician Alan Turing laid the conceptual groundwork for intelligent machines. His theoretical Turing machine, described in 1936, was a thought experiment designed to demonstrate that a machine could simulate any human-held notion of computation. Turing’s ideas laid the foundation for the development of digital computers, and by extension, the field of AI.
In the following decades, computer scientists and mathematicians began to explore the idea of creating machines that could mimic human intelligence. The Dartmouth Workshop in 1956, often considered the birth of AI as a formal field, brought together pioneers like John McCarthy, Marvin Minsky, Nathaniel Rochester, and Claude Shannon. They aimed to create machines that could simulate human problem-solving and decision-making processes.
The AI Winter and Resurgence
Despite early optimism, AI research faced numerous challenges during the late 1960s and 1970s. Progress was slow, and the initial enthusiasm waned, leading to what’s known as the “AI winter.” Funding for AI research became scarce as it failed to deliver on its lofty promises.
However, AI didn’t disappear; it just evolved quietly in the background. Expert systems, which relied on rule-based logic to simulate human expertise, found applications in fields like medicine and finance. Neural networks, inspired by the structure of the human brain, also continued to develop.
The resurgence of AI in the 21st century can be attributed to several factors:
- Big Data: The exponential growth of digital data provided the fuel needed to train and improve AI algorithms. More data meant better models.
- Computational Power: Advances in computing hardware, including Graphics Processing Units (GPUs), made it possible to train complex AI models more efficiently.
- Deep Learning: Deep neural networks, with many layers of interconnected nodes, proved to be highly effective in tasks like image recognition and natural language processing.
- Algorithmic Breakthroughs: Innovations in machine learning algorithms, such as backpropagation and stochastic gradient descent, improved the training and optimization of neural networks.
- Open Source and Collaboration: The open-source movement and a culture of collaboration enabled researchers worldwide to build on each other’s work, accelerating progress.
Transformative Applications
Today, AI has transcended theoretical concepts and academic discussions to become an integral part of our daily lives:
- Voice Assistants: Siri, Alexa, and Google Assistant have become household names, offering voice-activated assistance for tasks ranging from setting reminders to answering questions.
- Autonomous Vehicles: Companies like Tesla are pushing the boundaries of autonomous driving, leveraging AI for real-time decision-making on the road.
- Healthcare: AI is transforming healthcare through applications like disease diagnosis, drug discovery, and personalized treatment plans.
- Finance: Algorithmic trading, fraud detection, and risk assessment rely heavily on AI to process vast amounts of financial data.
- Customer Service: Chatbots and virtual assistants are revolutionizing customer service by providing 24/7 support and instant responses.
- Entertainment: AI is used to recommend movies, songs, and products based on user preferences, shaping our entertainment choices.
- Manufacturing: AI-driven robots and automation are optimizing manufacturing processes, improving efficiency and quality.
- Education: Personalized learning platforms use AI to tailor educational content to individual student needs.
- Natural Language Processing: AI-powered language models can generate human-like text, revolutionizing content creation and translation.
The evolution of AI from theoretical concepts to practical applications has been remarkable. Yet, as AI continues to advance, it paves the way for another groundbreaking technology: quantum computing.
Quantum Computing: The Frontier of Computation
Quantum computing, often described as the next quantum leap in computational power, represents a radical departure from classical computing. While classical computers rely on bits to process information as either 0 or 1, quantum computers use quantum bits, or qubits, which can exist in multiple states simultaneously due to the principles of superposition and entanglement.
The Birth of Quantum Mechanics
Quantum computing finds its origins in the early 20th century with the development of quantum mechanics. Pioneers like Max Planck, Albert Einstein, Niels Bohr, and Erwin Schrödinger were at the forefront of unraveling the mysterious behavior of particles at the quantum level. Their work laid the foundation for a new understanding of the fundamental building blocks of the universe.
The Birth of Quantum Computing: Richard Feynman and David Deutsch
The idea of quantum computing as a concept dates back to 1981 when physicist Richard Feynman proposed the concept of a quantum computer to simulate quantum systems more efficiently than classical computers. Feynman’s insight was a critical step, but it was David Deutsch’s work in the mid-1980s that solidified the theoretical basis for quantum computing. Deutsch’s algorithms showed that quantum computers could solve problems exponentially faster than classical computers.
Building Quantum Bits
Quantum bits, or qubits, are the heart of quantum computing. Unlike classical bits, which are either 0 or 1, qubits can exist in a superposition of states, allowing them to perform multiple calculations simultaneously. This inherent parallelism forms the basis of quantum computing’s power.
Qubits can be realized using various physical systems, including:
- Superconducting Circuits: Qubits are implemented using tiny superconducting electrical circuits cooled to extremely low temperatures.
- Trapped Ions: Individual ions are trapped and manipulated using electromagnetic fields to serve as qubits.
- Topological Qubits: These qubits are based on exotic states of matter known as topological superconductors.
- Photonic Qubits: Qubits are encoded in the properties of photons, particles of light.
- Nuclear Magnetic Resonance (NMR): Liquid-state NMR uses the nuclear spins of molecules as qubits.
- Silicon Spin Qubits: Quantum dots in silicon are manipulated to serve as qubits.
- Majorana Fermions: These exotic particles, if they exist, could be used as qubits.
Each approach has its advantages and challenges, and researchers are actively exploring which technology will be most practical for building large-scale quantum computers.